|
ACCESS THE FULL ARTICLE
No SPIE Account? Create one
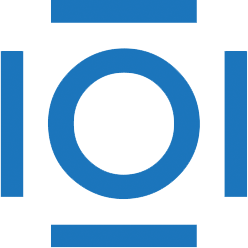
CITATIONS
Cited by 3 scholarly publications.
Principal component analysis
Optical engineering
Heart
Breast
Databases
Data modeling
Machine vision