|
ACCESS THE FULL ARTICLE
No SPIE Account? Create one
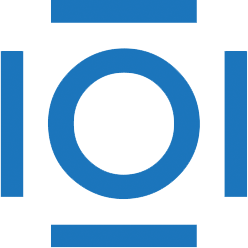
CITATIONS
Cited by 3 scholarly publications.
Receivers
Data modeling
Transducers
Photoacoustic spectroscopy
Acoustics
Interference (communication)
Convolutional neural networks