|
ACCESS THE FULL ARTICLE
No SPIE Account? Create one
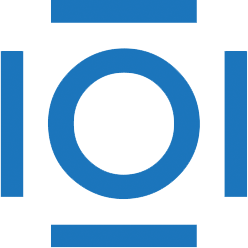
CITATIONS
Cited by 5 scholarly publications.
Eye
Data modeling
Convolutional neural networks
Feature extraction
Performance modeling
Eye models
Optic nerve