First, we propose a method to identify the emitters that provide reconstructions with minimal expected uncertainties. Using a Bayesian approach, model uncertainties and resolution can be quantified with the trace of the posterior covariance. By linearizing the wave equation, we can compute the posterior covariance using the inverse of the Gauss-Newton approximation of the Hessian. Furthermore, this posterior is independent of the breast model and the experimental data, thus enabling pre-acquisition experimental optimization. Then, for the post-acquisition inversion, we present an approach to select a subsample of sources that accurately approximates the full gradient direction in each iteration. We control the convergence of the angular differences between consecutive gradient directions by randomly adding new emitters into the subsample. We present synthetic studies in 2D and 3D that consider a ring-shaped and a semi-ellipsoidal scanning device, respectively. Numerical results suggest that the provided methods have the potential to identify redundancies from the corresponding cost-benefit curves. Furthermore, the gradient direction rapidly converges to the direction of the full gradient, which appears to be independent of the model and the emitter locations. |
ACCESS THE FULL ARTICLE
No SPIE Account? Create one
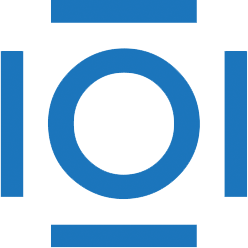
CITATIONS
Cited by 8 scholarly publications.
Transducers
Data modeling
Breast
Tomography
Inverse problems
Ultrasonography
Quality measurement