|
ACCESS THE FULL ARTICLE
No SPIE Account? Create one
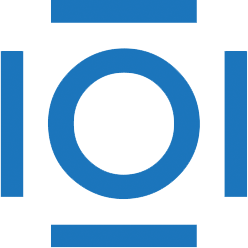
CITATIONS
Cited by 1 patent.
Convolution
Feature extraction
Convolutional neural networks
Image classification
Data acquisition
Hyperspectral imaging
3D image processing