|
ACCESS THE FULL ARTICLE
No SPIE Account? Create one
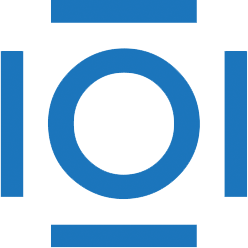
CITATIONS
Cited by 3 scholarly publications.
Point spread functions
Wavefronts
Machine learning
Monte Carlo methods
Data modeling
Phase retrieval
James Webb Space Telescope