|
ACCESS THE FULL ARTICLE
No SPIE Account? Create one
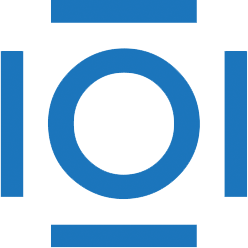
CITATIONS
Cited by 1 scholarly publication.
Computer aided diagnosis and therapy
Feature extraction
Computer-aided diagnosis
Calcium
Chest
Computing systems
3D image processing