|
ACCESS THE FULL ARTICLE
No SPIE Account? Create one
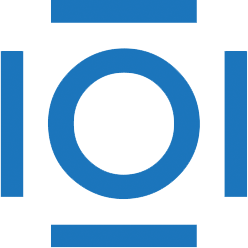
CITATIONS
Cited by 1 scholarly publication.
Synthetic aperture radar
Image classification
Machine learning
Network architectures
Algorithm development
Detection and tracking algorithms
Image processing