|
ACCESS THE FULL ARTICLE
No SPIE Account? Create one
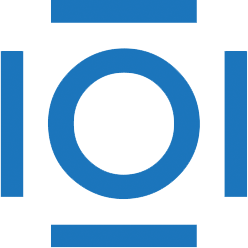
CITATIONS
Cited by 1 scholarly publication.
Cancer
Kidney
Computed tomography
Visualization
Convolutional neural networks
Image classification
Medical imaging