|
ACCESS THE FULL ARTICLE
No SPIE Account? Create one
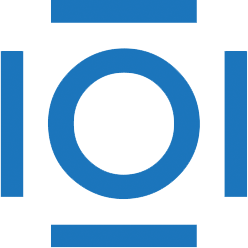
CITATIONS
Cited by 4 scholarly publications.
Network architectures
Optical character recognition
Nonlinear image processing
Feature extraction
Error analysis
Ions
Model-based design