|
ACCESS THE FULL ARTICLE
No SPIE Account? Create one
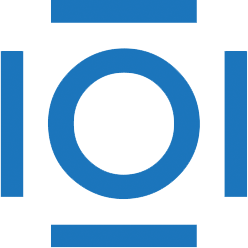
CITATIONS
Cited by 2 scholarly publications.
Image processing
Binary data
Clocks
Image classification
Visual communications
Pattern recognition
Very large scale integration