|
ACCESS THE FULL ARTICLE
No SPIE Account? Create one
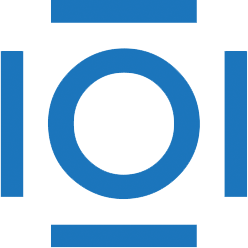
CITATIONS
Cited by 4 scholarly publications.
Fuzzy logic
Classification systems
Distance measurement
Neural networks
Artificial neural networks
Neurons
Associative arrays