|
ACCESS THE FULL ARTICLE
No SPIE Account? Create one
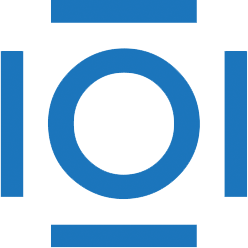
CITATIONS
Cited by 6 scholarly publications.
Radar
Neural networks
Artificial neural networks
Brain
Network architectures
Pattern recognition
Image classification