|
ACCESS THE FULL ARTICLE
No SPIE Account? Create one
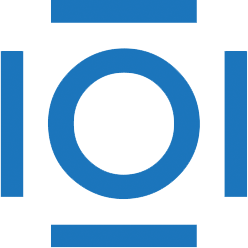
CITATIONS
Cited by 7 scholarly publications.
Error analysis
Silicon
Information operations
Artificial neural networks
Neural networks
Pattern recognition
Optical character recognition