|
ACCESS THE FULL ARTICLE
No SPIE Account? Create one
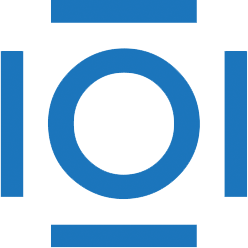
CITATIONS
Cited by 3 scholarly publications.
Detection and tracking algorithms
Analog electronics
Algorithm development
Artificial neural networks
Computing systems
Lithium
Quantization