|
ACCESS THE FULL ARTICLE
No SPIE Account? Create one
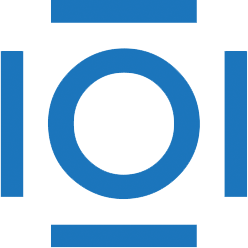
CITATIONS
Cited by 1 scholarly publication.
Wavefronts
Signal to noise ratio
Neural networks
Wavefront sensors
Artificial neural networks
Computer simulations
Sensors