|
ACCESS THE FULL ARTICLE
No SPIE Account? Create one
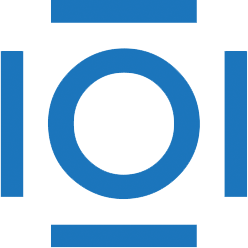
CITATIONS
Cited by 1 scholarly publication.
Neurons
Neural networks
Binary data
Algorithms
Matrices
Zirconium
Astatine