|
ACCESS THE FULL ARTICLE
No SPIE Account? Create one
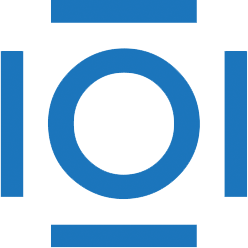
CITATIONS
Cited by 13 scholarly publications.
Process modeling
Systems modeling
Error analysis
Sensors
Filtering (signal processing)
Data modeling
Motion models