|
ACCESS THE FULL ARTICLE
No SPIE Account? Create one
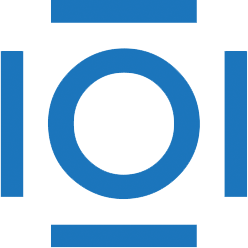
CITATIONS
Cited by 3 scholarly publications.
Databases
Synthetic aperture radar
Polarization
Data modeling
Neural networks
Model-based design
Image classification