|
ACCESS THE FULL ARTICLE
No SPIE Account? Create one
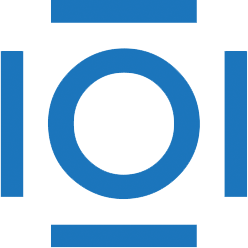
CITATIONS
Cited by 2 scholarly publications.
Satellites
Pattern recognition
Neural networks
3D displays
3D modeling
Detection and tracking algorithms
Satellite imaging