|
ACCESS THE FULL ARTICLE
No SPIE Account? Create one
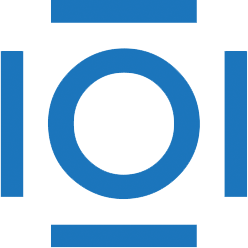
CITATIONS
Cited by 1 scholarly publication.
Transmitters
LIDAR
Signal to noise ratio
Data modeling
Statistical analysis
Detection and tracking algorithms
Absorption