|
ACCESS THE FULL ARTICLE
No SPIE Account? Create one
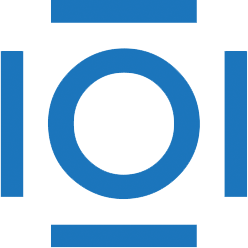
CITATIONS
Cited by 123 scholarly publications.
Independent component analysis
Minerals
Image sensors
Statistical analysis
Data analysis
Hyperspectral imaging