|
ACCESS THE FULL ARTICLE
No SPIE Account? Create one
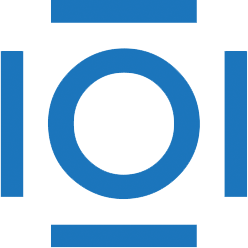
CITATIONS
Cited by 3 scholarly publications.
Principal component analysis
Neural networks
Algorithm development
MATLAB
Error analysis
Evolutionary algorithms
Neurons