|
ACCESS THE FULL ARTICLE
No SPIE Account? Create one
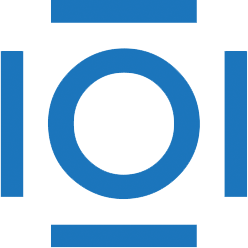
CITATIONS
Cited by 5 scholarly publications.
Fuzzy logic
Data fusion
Sensors
Data processing
Probability theory
Data modeling
Surveillance