|
ACCESS THE FULL ARTICLE
No SPIE Account? Create one
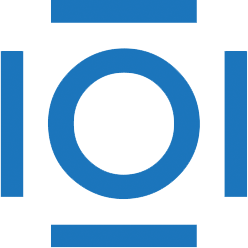
CITATIONS
Cited by 5 scholarly publications.
Sensors
Signal detection
Acoustic emission
Principal component analysis
Neural networks
Data centers
Metals