|
ACCESS THE FULL ARTICLE
No SPIE Account? Create one
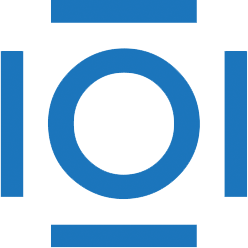
CITATIONS
Cited by 3 scholarly publications.
Neural networks
Performance modeling
C++
Systems modeling
Networks
Sensors
Analytical research