|
ACCESS THE FULL ARTICLE
No SPIE Account? Create one
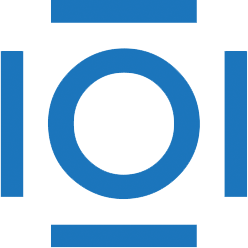
CITATIONS
Cited by 7 scholarly publications.
Statistical modeling
Mathematical modeling
Stochastic processes
Principal component analysis
Image filtering
Monte Carlo methods
Feature extraction