|
ACCESS THE FULL ARTICLE
No SPIE Account? Create one
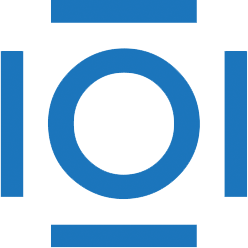
CITATIONS
Cited by 2 scholarly publications.
Target detection
Statistical modeling
Visual process modeling
Bayesian inference
Probability theory
Image analysis
Statistical analysis