|
ACCESS THE FULL ARTICLE
No SPIE Account? Create one
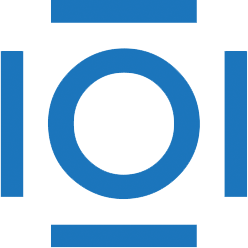
CITATIONS
Cited by 4 scholarly publications.
Radar
Image classification
Synthetic aperture radar
Reflectivity
Speckle
Stochastic processes
Device simulation