|
ACCESS THE FULL ARTICLE
No SPIE Account? Create one
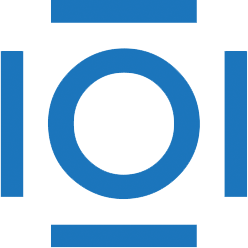
CITATIONS
Cited by 1 scholarly publication.
Autoregressive models
Image filtering
Image processing
Image compression
Data modeling
Expectation maximization algorithms
Target detection