|
ACCESS THE FULL ARTICLE
No SPIE Account? Create one
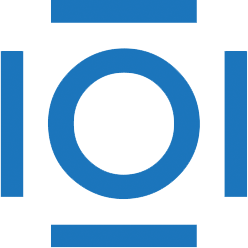
CITATIONS
Cited by 1 scholarly publication.
Mining
General packet radio service
Land mines
Sensors
Data modeling
Detection and tracking algorithms
Expectation maximization algorithms