|
ACCESS THE FULL ARTICLE
No SPIE Account? Create one
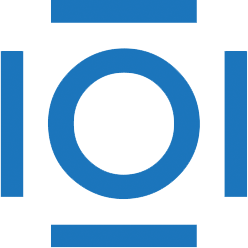
CITATIONS
Cited by 9 scholarly publications.
Tumors
Leukemia
Colon
Feature selection
Computer generated holography
Diagnostics
Cancer