|
ACCESS THE FULL ARTICLE
No SPIE Account? Create one
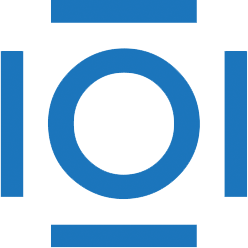
CITATIONS
Cited by 47 scholarly publications.
Video
Databases
Data modeling
Model-based design
Multimedia
Distance measurement
Image segmentation