|
ACCESS THE FULL ARTICLE
No SPIE Account? Create one
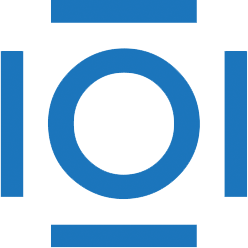
CITATIONS
Cited by 3 scholarly publications.
Remote sensing
Data modeling
Data conversion
Agriculture
Geographic information systems
Vegetation
Data acquisition