|
ACCESS THE FULL ARTICLE
No SPIE Account? Create one
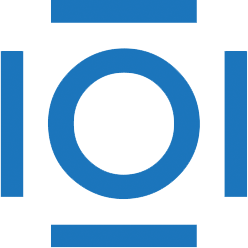
CITATIONS
Cited by 2 scholarly publications.
Hyperspectral imaging
Sensors
Absorption
Air contamination
Principal component analysis
Reflectivity
Signal to noise ratio