|
ACCESS THE FULL ARTICLE
No SPIE Account? Create one
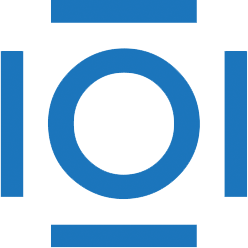
CITATIONS
Cited by 2 scholarly publications.
Deconvolution
Denoising
Point spread functions
Charge-coupled devices
Reconstruction algorithms
Algorithm development
Image restoration