|
ACCESS THE FULL ARTICLE
No SPIE Account? Create one
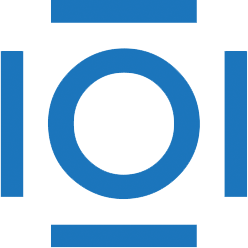
CITATIONS
Cited by 5 scholarly publications.
Ultrasonography
Tomography
Image analysis
Image classification
Machine learning
Scattering
Surface plasmons