|
ACCESS THE FULL ARTICLE
No SPIE Account? Create one
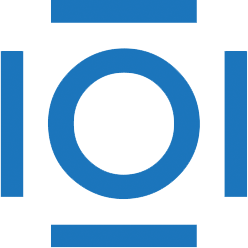
CITATIONS
Cited by 1 scholarly publication.
LIDAR
Data modeling
Data fusion
Error analysis
Filtering (signal processing)
Motion models
Algorithm development