|
ACCESS THE FULL ARTICLE
No SPIE Account? Create one
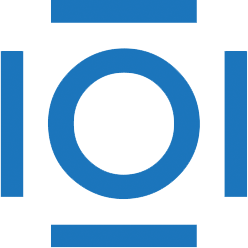
CITATIONS
Cited by 37 scholarly publications and 1 patent.
Cameras
Video
Video processing
Radar
Video surveillance
Artificial intelligence
Data modeling