|
ACCESS THE FULL ARTICLE
No SPIE Account? Create one
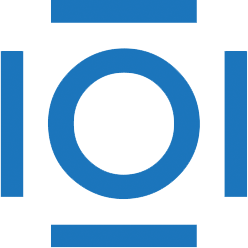
CITATIONS
Cited by 4 scholarly publications.
Sensors
Sensor networks
Data compression
Data centers
Data communications
Data fusion
Algorithm development