|
ACCESS THE FULL ARTICLE
No SPIE Account? Create one
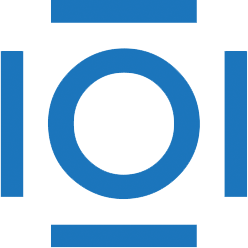
CITATIONS
Cited by 2 scholarly publications.
Image segmentation
Tissues
Expectation maximization algorithms
Photovoltaics
Colon
Bone
Image processing algorithms and systems