|
ACCESS THE FULL ARTICLE
No SPIE Account? Create one
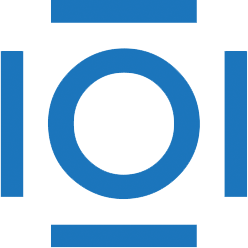
CITATIONS
Cited by 9 scholarly publications.
Calcium
Neural networks
Thalamus
Axons
Brain
Dendrites
Mathematical modeling