|
ACCESS THE FULL ARTICLE
No SPIE Account? Create one
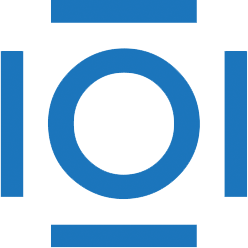
CITATIONS
Cited by 5 scholarly publications.
Discrete wavelet transforms
Expectation maximization algorithms
Magnetorheological finishing
Image fusion
Wavelets
Wavelet transforms
Image processing