|
ACCESS THE FULL ARTICLE
No SPIE Account? Create one
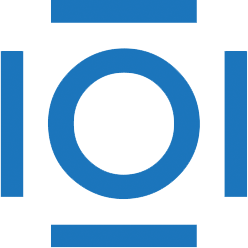
CITATIONS
Cited by 2 scholarly publications.
Neurons
Data modeling
Space operations
Neural networks
Artificial neural networks
Autoregressive models
Performance modeling