|
ACCESS THE FULL ARTICLE
No SPIE Account? Create one
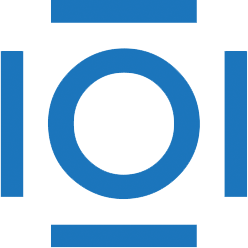
CITATIONS
Cited by 7 scholarly publications.
Diffusion
Hyperspectral imaging
Image classification
Denoising
Image processing
Tellurium
Image segmentation