|
ACCESS THE FULL ARTICLE
No SPIE Account? Create one
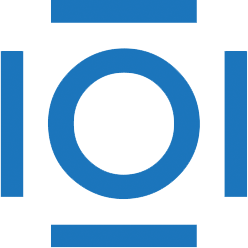
CITATIONS
Cited by 2 scholarly publications.
Data modeling
Sensors
Neural networks
Analytical research
Complex systems
Systems modeling
Process modeling