|
ACCESS THE FULL ARTICLE
No SPIE Account? Create one
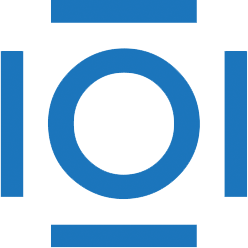
CITATIONS
Cited by 3 scholarly publications.
Inspection
Statistical analysis
Image segmentation
Image filtering
Defect detection
Wavelets
Image analysis