|
ACCESS THE FULL ARTICLE
No SPIE Account? Create one
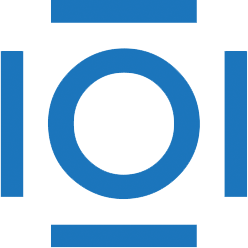
CITATIONS
Cited by 13 scholarly publications.
Image segmentation
Data modeling
Principal component analysis
Optical alignment
Binary data
Shape analysis
Cardiovascular magnetic resonance imaging