|
ACCESS THE FULL ARTICLE
No SPIE Account? Create one
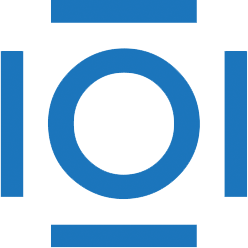
CITATIONS
Cited by 1 scholarly publication.
Compressed sensing
Signal to noise ratio
Brain
Magnetic resonance imaging
In vitro testing
Computer simulations
Reconstruction algorithms