|
ACCESS THE FULL ARTICLE
No SPIE Account? Create one
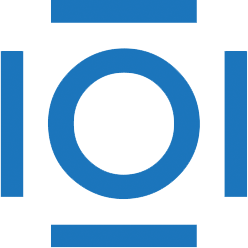
CITATIONS
Cited by 9 scholarly publications.
Image classification
Hyperspectral imaging
Remote sensing
Vegetation
Genetic algorithms
Statistical analysis
Classification systems